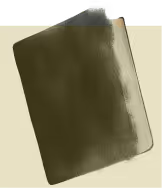
Announcing Our $50M Series A to Advance AI Interpretability Research
Funding from Menlo Ventures powers our mission to decode the neurons of AI models, reshaping how they're understood and designed
Published
April 17, 2025
Today, we're excited to announce a $50 million Series A funding round led by Menlo Ventures with participation from Lightspeed Venture Partners, Anthropic, B Capital, Work-Bench, Wing, South Park Commons, and other notable investors. This funding, which comes less than one year after our founding, will support the expansion of our research initiatives and the development of our flagship interpretability platform, Ember, in partnership with customers.
“AI models are notoriously nondeterministic black boxes,” said Deedy Das, investor at Menlo Ventures. “Goodfire’s world-class team—drawn from OpenAI and Google DeepMind—is cracking open that box to help enterprises truly understand, guide, and control their AI systems.”
Despite remarkable advances in AI, even leading researchers have little idea of how neural networks truly function. This knowledge gap makes neural networks difficult to engineer, prone to unpredictable failures, and increasingly risky to deploy as these powerful systems become harder to guide and understand.
“Nobody understands the mechanisms by which AI models fail, so no one knows how to fix them,” said Eric Ho, co-founder and CEO of Goodfire. “Our vision is to build tools to make neural networks easy to understand, design, and fix from the inside out. This technology is critical for building the next frontier of safe and powerful foundation models.”
To solve this critical problem, we are investing significantly in mechanistic interpretability research – the relatively nascent science of reverse engineering neural networks and translating those insights into a universal, model-agnostic platform. Known as Ember, Goodfire’s platform decodes the neurons inside of an AI model to give direct, programmable access to its internal thoughts. By moving beyond black-box inputs and outputs, Ember unlocks entirely new ways to apply, train, and align AI models — allowing users to discover new knowledge hidden in their model, precisely shape its behaviors, and improve its performance.
"As AI capabilities advance, our ability to understand these systems must keep pace. Our investment in Goodfire reflects our belief that mechanistic interpretability is among the best bets to help us transform black-box neural networks into understandable, steerable systems—a critical foundation for the responsible development of powerful AI," said Dario Amodei, CEO and Co-Founder of Anthropic.
Looking ahead, we are accelerating interpretability research through targeted initiatives with frontier model developers. By closely partnering with industry innovators, Goodfire will rapidly enhance and solidify the application of interpretability research. “Partnering with Goodfire has been instrumental in unlocking deeper insights from Evo 2, our DNA foundation model,” said Patrick Hsu, co-founder of Arc Institute – one of Goodfire’s earliest collaborators. “Their interpretability tools have enabled us to extract novel biological concepts that are accelerating our scientific discovery process.”
We are planning to release additional research previews, highlighting state-of-the-art interpretability techniques across diverse fields such as image processing, advanced reasoning language models, and scientific modeling. These efforts promise to reveal new scientific insights and fundamentally reshape our understanding of how we can interact with and leverage AI models.
Our team unites top AI interpretability researchers and experienced startup operators from organizations like OpenAI and Google DeepMind. Our researchers helped found the field of mechanistic interpretability, authoring three of the most-cited papers and pioneering advancements like Sparse Autoencoders (SAEs) for feature discovery, auto-interpretability frameworks, and revealing the hidden knowledge in AI models.